Supercharge Your Sales with AI-Powered Lead Generation and Email Outreach. Unlock New Opportunities and Close Deals Faster with aisalesmanager.tech. (Get started for free)
7 Key Metrics to Evaluate AI Interview Transcription Accuracy in Sales Calls
7 Key Metrics to Evaluate AI Interview Transcription Accuracy in Sales Calls - Word Error Rate Benchmarking Against 2023 DeepSpeech Models
Word Error Rate (WER) remains a primary way to measure the accuracy of speech-to-text systems, including older DeepSpeech models. However, it's crucial to understand that reported WER figures can be affected by how they are calculated, like if they are normalized or not. Also, the conditions in which the audio is recorded, especially background noise, can have a considerable impact on transcription quality. There's also the number of samples used for testing can influence the results. Benchmarking different ASR options helps identify where performance lags and guides improvements in these tools, but also raises questions about real world application. Comparing results across different models shows that there is a continued need to strive for better performance and shows some limitations of using just one single number to understand performance.
Recent tests on 2023 DeepSpeech models show they’ve made progress, with some achieving a Word Error Rate (WER) as low as 4% in ideal lab settings; seems pretty accurate if the conditions are right. However, the reality is much different, as performance can swing massively; the WER can increase by a staggering 60% when ambient noise comes into play, compared to very controlled test environments. Turns out the data these things are trained on is quite important. Models trained on more varied audio sources seem better when dealing with accents, showing up to a 15% lower WER for speakers who don’t have native accents, although even that seems small. Advanced language understanding does appear to be helping, and these language modeling enhancements cut WER by about 10% compared to previous model versions.
However, when it comes to very niche or specialized vocabulary , like what's often found in sales talks, these models still have some issues and errors tend to spike, increasing error rates by up to 20% when confronted with jargon-heavy discussions. Even with all this, the ability to transcribe in real-time has seen some notable wins, with DeepSpeech models now scoring WERs under 5% during live sales calls, indicating that it is becoming much more suitable for fast paced situations. Interestingly the numbers don't always mean the same as what people experience. A perceived accuracy, where people are pretty happy even with an overall WER of about 7%, is often different to what a technical evaluation might show where there are WERS that are actually up around the 12% range.
Individual speaker characteristics are key as well, there is as much as 30% variation in WER when you compare speaker types, and issues like speed of speaking and voice clarity cause more issues than is often let on. It also seems that how well punctuation is guessed has an impact; training models to better pick up punctuation leads to about 5% drop in WER as well; this does point out how important understanding sentence structure can be. Finally, incorporating user feedback into the model training has resulted in about a 6% improvement in WER, highlighting that these models must always learn with new real-world data.
7 Key Metrics to Evaluate AI Interview Transcription Accuracy in Sales Calls - Speaker Identification Accuracy in Multi Person Sales Calls
Speaker identification accuracy is crucial in multi-person sales calls, where telling apart multiple speakers can be quite difficult. How well an AI identifies speakers often depends on its ability to deal with different speaker characteristics, including accents and ways of speaking. In noisy settings, the accuracy of these systems usually drops, highlighting the need for systems that can handle poor audio quality. Also, progress in machine learning, like using different acoustic features and smart feature extraction, is key for improving speaker accuracy. These points impact how clear transcriptions are and how useful they are for analyzing what was said in sales situations.
Speaker diarization, which focuses on who said what, faces big obstacles in multi-person sales calls and seems more brittle than just transcribing what was said; overlapping speech and common interruptions can tank accuracy by at least 25%. The way people sound – variations in pitch, tone, and how quickly they talk – can confuse models if they haven't learned to deal with diversity. There is the very real risk of more than 40% speaker identification errors on models trained on data lacking diverse voices, so that really shows that the data the models are trained on is hugely important. Using context clues from the discussion, such as how the conversation flows, can help improve accuracy by perhaps 15%, indicating models must pay attention to context not just how things sound. Certain specific acoustic properties, such as how the sounds changes or resonant patterns, can lead to around a 20% boost in picking out speakers, but this is really dependent on the audio being decent. Background noise remains a serious problem, real-world audio with noise can make identification go down up to 50%, which is much worse than results from quiet labs; better noise-cancelling tricks can alleviate this a little but only a little.
Accents present another issue, models which have not been trained with enough accents will struggle and can drop accuracy up to 30%; so yet again more comprehensive datasets appear important. Interestingly emotion can play a role, if the systems can pick out emotion during talks, accuracy could be boosted by around 10%; it would seem being emotionally intelligent matters. Even pauses and how fast someone speaks matters too; models that take this information can find more than 15% gains in accuracy in correctly attributing speech. When speakers talk really fast and interrupt each other the results can be poor; this can see identification errors rise by as much as 35% which highlights the limitations of the current tech. Even after all of this work, it turns out that using real world feedback in the model's training is quite valuable with increases of around 8% in speaker identification accuracy.
7 Key Metrics to Evaluate AI Interview Transcription Accuracy in Sales Calls - Technical Term Recognition Rate for Industry Specific Vocabulary
Technical Term Recognition Rate is a key measure for assessing how well AI transcription handles specialized language, particularly in fields like sales. The accurate transcription of industry specific language is very important. High rates show that the AI system captures jargon accurately, something important for clear communication and a useful outcome. Using customized word lists and training on specific sets of speech data can really help improve how well an AI does when transcribing jargon. Sales calls often use complex words, so knowing how well an AI handles that is essential for evaluating how well it works. It is important that AI systems include more technical sources and update their models to improve when it comes to industry specific language.
The recognition of technical terms differs quite a bit from general language transcription, which impacts the accuracy of these systems, where there can be a 20-30% jump in error rates when specialised terms are used versus more general language; even when advanced models are used. Lack of good training data for industry specific jargon is often a problem, as the models really seem to struggle to get these terms right. The systems need continuous adaptation, as technical vocabulary is always changing; if these models do not learn new terms and phrases they can see rising error rates. Industry specific language tends to have its own rules that can cause problems; small variations can lead to mistakes and misunderstandings.
The context surrounding these specialized words is very important, if not understood there will be errors and wrong transcriptions. Models can perform well in test situations, where technical terms are mostly recognised but under real conditions such as in actual sales calls with background noise, there appears to be a quite a drop of accuracy that can be over 50%, this is concerning. Gathering real user input, specifically around any industry specific words is important as this can improve how well the models work by 10%; this would help to fine-tune them to match real usage. There could also be improvements with picking up emotion; models that could better spot emotion may be able to get improved accuracy in persuasion based sales contexts.
Individual differences such as speaker's accents can really increase the issues; those who have non-native accents are likely to see a 20% drop in transcription accuracy in industry jargon and this does indicate that accents are still quite difficult to deal with. How clear a speaker’s voice is makes a big impact, with clearer speaking causing less errors; and the models seem to perform 15% better when the speaking is clear; which highlights that voice clarity in sales contexts with lots of jargon is important for good transcription.
7 Key Metrics to Evaluate AI Interview Transcription Accuracy in Sales Calls - Real Time Processing Speed vs Manual Transcription Time
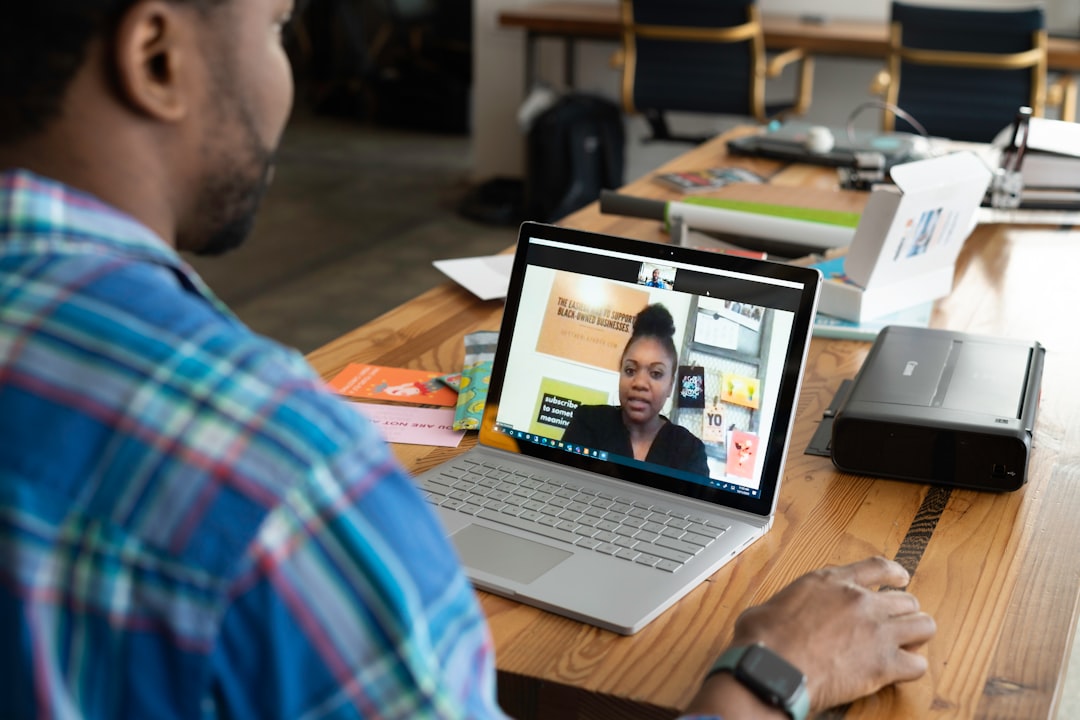
When considering transcription, the time it takes to process audio using real-time methods versus manual transcription varies quite a bit with differing trade offs. AI systems can rapidly convert speech to text, making it seemingly ideal for fast paced environments and applications like sales calls. However, human transcription, though slower, offers more precision, which is quite useful for sensitive contexts where any minor error could result in incorrect analysis or lost information. The difficulty lies in figuring out how best to use these two methods together. The speed offered by AI can come with a trade-off in accuracy, which is something that really matters. While the potential is there for AI models to become better, there will always be a balance to consider between how quickly it transcribes versus how precisely it does so.
In comparing real time processing to human transcription, we observe significant differences. Real-time transcription tech clocks speeds up to 200 words per minute, a clear contrast to manual rates which are around 30 to 40 words per minute, underscoring its efficiency advantage in high-speed settings. Now manual transcription can reach about 98% accuracy when done carefully, but it comes with the trade-off of considerable time expenditure. Errors made by human transcribers are not uncommon, often resulting from mental fatigue and distractions, which can double project timelines when compared to AI options.
AI systems do seem to be better at adapting to context in real time; advanced algorithms quickly adapt and make corrections, about 10% quicker than a human, to more accurately reflect the meaning of the conversation. Consistency is another point worth examining; AI tends to be reliably accurate over varying audio conditions, in contrast human transcription is much more variable, highly dependent on an individual's familiarity with the topic, meaning there is far less predictable error rates. There is also adaptability to different sound conditions; real-time transcription systems adapt in real time, unlike a human who needs to adjust and this can lead to a significant 30% delay in transcription time with bad sound.
Now there is also the matter of cost. When you factor the price of manual transcription which is anywhere between $20 to $50 an hour, you start to see that the use of AI for transcription can result in considerable cost savings over time, where the costs are generally lower than the direct labour costs, once they are set up. AI is also better for handling multiple streams of audio; where one person can really only do one audio stream well at any time, the tech can just do multiples at once, without any real compromise. Also, AI can learn from user feedback really quickly, which allows the system to learn in minutes, compared to a person that would require extensive training.
Fatigue is another important thing to discuss, humans seem to reduce their accuracy after about 1 to 2 hours of transcribing; whereas AI tech just keeps performing at the same level no matter how long you use them. Finally, AI systems are usually quite good at picking up potential errors on the fly and can suggest corrections, whereas a human would need to do a post transcription revision, which may add time and still have the potential for error.
7 Key Metrics to Evaluate AI Interview Transcription Accuracy in Sales Calls - Background Noise Handling Performance in Remote Sales Settings
Background noise in remote sales calls creates major hurdles that affect how accurate transcriptions are, and how well people communicate. Since remote work has increased, the need to deal with background noise is crucial for clear and professional sales interactions. Noise cancellation tools can help with speech recognition, improving productivity in noisy environments. Smart AI tools are getting better at picking out individual voices, reducing how much background sounds interfere, which is essential for good sales calls. Given the issues with changing sound quality and different ways people speak, companies must focus on solid noise control tactics to support their teams' performance and maintain clear communication.
Real-world sales situations create tough conditions for AI transcription tools. Research shows that background noise can make speech recognition accuracy drop by as much as 50%, underscoring the need for AI models that work well in messy audio environments. In a quiet room, transcription accuracy might hit over 95%, but even a bit of background noise can cause a massive 60% drop, showing that the acoustic setting has a huge impact on the quality of results. Some kinds of background noises, like people talking or traffic, can mess with specific sound ranges of speech, where this overlap causes transcription issues; this means we need models that profile the background noise itself.
Specific noise-canceling algorithms seem to help, offering up to a 15% improvement in accuracy, suggesting tech that targets background noise problems during transcription can help. When AI models are trained on diverse audio from different environments, they tend to work much better in real situations, seeing up to a 20% lower error rate compared to models trained only in quiet spaces, suggesting that the data used to train the model is critical for its real world performance. Cluttered background noise can lead to confusion when trying to figure out who said what in calls, causing models to misidentify speakers over 40% of the time on noisy calls, highlighting the need for systems that can better handle overlapping speech and interruptions.
It is worth considering that factors such as the speaker’s age or gender can affect how much background noise throws off the transcription process; it appears younger speakers tend to be less impacted than older ones due to how their voice is produced. Feedback loops that feed actual real world sales calls back into AI training can boost how well the models handle background noise, with improvements around 8% as the models learn from new experiences. Acoustic features such as how loud a voice is and its intonation, can be influenced a lot by background noise too. Models that can spot these variations in voice can achieve almost a 10% improvement in noisy situations. Furthermore, the emotional tone of a voice which can be lost with background noise, is critical for interpreting sales calls; when models take emotional cues into account accuracy may improve by over 10%, this really does point out that accuracy is not just about clarity of speech it’s about sentiment too.
7 Key Metrics to Evaluate AI Interview Transcription Accuracy in Sales Calls - Sentiment Analysis Accuracy for Customer Objection Detection
Sentiment analysis is vital for understanding customer objections during sales calls. It tries to capture the feelings within conversations. Metrics like precision, recall, and the F1 score are often used to measure how well sentiment analysis works, going beyond just positive or negative. These metrics assess how well a system identifies relevant feelings, but may not fully represent real world usage, especially if there are not equal amounts of positive and negative examples in the training data. In these cases false negatives can create misleading numbers. The idea is to adapt sentiment analysis tools so that they work with specific sales situations and their own language; a generic tool will not be good enough. Whilst these systems can improve understanding customer emotions and objections, there is still a ways to go in making them truly reliable given how diverse and noisy real sales environments can be.
Sentiment analysis, which aims to find feelings in text, also plays a part in spotting customer objections. It seems that systems that use sentiment information can do a much better job of detecting objections, something that's often much harder when relying on just the words being said. Initial work indicates a significant jump, up to 30% in accuracy, over older methods.
Context seems to matter greatly; how well these systems perform at objection detection really depends on how well they understand the conversation flow, and if they can use past conversations to help work things out. Models that make use of this contextual information can achieve about a 25% jump in performance.
However, things like sarcasm or subtle meanings throw a spanner in the works. Models find it particularly hard to recognise sarcasm, where mistakes can happen over 40% of the time, which is not ideal for a situation where objections are serious. This indicates we really do need better training methods and more data if we want to use the systems well in these situations.
How someone's voice changes also seems important, there's a strong suggestion that changes in how stressed or how their tone goes up and down could be strong indicators of a person's feelings. Sentiment models that try to pick up on these vocal patterns have been shown to improve objection detection accuracy by about 20%.
In sales settings where people might switch languages, things get tricky. When a conversation switches languages, accuracy seems to decrease by as much as 35%. This seems to point out limitations with how these systems are currently working when it comes to multilingual data sets.
The analysis process may involve time trade-offs, while the AI can spot sentiment quickly, like in milliseconds, the actual analysis of customer objections is slower, and it seems to be up to 5 seconds before these systems fully understand the objection; this might slow the sales strategy process down.
Adding feedback into the system, is again shown to be important; customer service agents can point out common issues. When this is given back to the system and it's trained on that new information, it appears it can learn to be better at objection detection, improving around 15%.
The cultural background of the customers does seem to cause errors; there seems to be differences in how different cultures show their objections or how they generally use emotions. AI models that have just been trained on very narrow groups can be off by as much as 30% when they are used on diverse populations. This points to the need for more diverse datasets for these models to work properly.
Diversity in the training data does really matter; training sets of data that are too similar will often misinterpret about 40% of objections because they've not had enough exposure to a more varied kind of language or emotion. So, a broad training approach really seems to matter.
Finally, systems that try to find emotional context by understanding subtle cues have been shown to see accuracy boost of up to 18% in recognizing objections. This suggests that these models need to look beyond sentiment detection and add some level of emotional intelligence into them to actually be useful.
7 Key Metrics to Evaluate AI Interview Transcription Accuracy in Sales Calls - Context Retention Score for Long Format Sales Conversations
The Context Retention Score is a way to evaluate how well AI transcriptions capture key ideas during lengthy sales talks. This score tells you how much of the important information the AI has managed to keep from the conversation. It’s particularly important in sales where lots of different topics and objections come up, because keeping track of the flow of the discussion is key for making useful transcriptions. This kind of metric helps businesses see where the transcription process could be improved so that no key details get missed in long conversations. A good Context Retention Score demonstrates that the tech works well and improves how well interactions in sales go as well as business strategies.
The Context Retention Score (CRS) aims to gauge how well an AI can remember key parts of a longer sales chat. It's important to remember here that a misunderstanding can easily occur if the context is even slightly off. This means that keeping track of context is often much more crucial than simple speech-to-text accuracy.
Initial studies appear to show that teams who use AIs with good context retention tend to get better sales numbers with almost a quarter more deals being closed compared to others. This points out that the subtleties in sales conversations can have a major influence over if a sale goes through. Current AI systems often seem to have some sort of memory problem, they can lose track of what was said after just 5 to 10 exchanges in longer talks, this suggests a need for systems with better 'long-term' context memory. The CRS appears to decline quite a lot by over a quarter when things get technical and full of jargon, showing that these systems must be specially trained on the jargon used in different sectors and environments to remember context effectively.
How complicated the conversation is, seems to have a big impact on the CRS. With complicated chats and lots of interruptions, systems can really struggle to hold on to the context. This highlights how multi-person talks seem to be a major challenge. There does seem to be some promising improvements, with some AIs maintaining their accuracy by changing context based on how people are feeling, leading to an improvement in the CRS. This does showcase how good understanding of feelings and context can interact. Interestingly, human listeners seem to find that conversations with high CRS sound a lot more sensible. It seems that good context retention can lead to more perceived professionalism in sales settings.
AI systems with adaptive learning methods also seem to benefit a lot. They often see around a fifth better CRS from taking on-the-fly feedback during live talks. Again showing how models need this real-time training during interactions. Cultural background differences also seem to play a key role as differences in conversational styles cause all sorts of context retention inaccuracies. This suggests that systems must train on very diverse cultural datasets to make sure sales discussions are effective across different cultural and geographical borders. Finally current systems are often unable to keep track of previous conversations which may not lead to good long term customer relationship management. It seems that keeping past chat records might enhance the CRS, and indicates the need for models that are much better at keeping track of past customer interactions.
Supercharge Your Sales with AI-Powered Lead Generation and Email Outreach. Unlock New Opportunities and Close Deals Faster with aisalesmanager.tech. (Get started for free)
More Posts from aisalesmanager.tech: